As magical as they may seem, face recognition systems don't just appear out of thin air. Instead, they are the product of countless hours of dedicated hard work, aimed at making the system just slightly better. Central to this process is the role of data, which is crucial in determining whether your system meets user expectations among various competing biometric authentication technologies.
This blog is a preview of a detailed report on the training data collection event for biometric detection AI models that Japan Computer Vision Corp. conducted in December 2023. The report will provide readers with a comprehensive understanding of the event, from the planning stage to the final engine construction, through firsthand accounts from the leaders of each section. In Part 1 of the report, we will focus on the planning stage and scenario creation.
Coming Up in This Series:
- Designing the Collection Tool and Pipeline
- Finding Participants and Locations
- Creating Scenarios
- Organizing the data
- Is manual reviewing needed?
- Completing the Dataset
- Why check again?
- Combining Datasets
- Creating Data Splits
- Conclusion: So long and thanks for all the data
1. Design of Collecting Tool and Pipeline
In this event, our goal is to collect about 100 images per person, with various situations, angles, and face accessories (e.g. glasses, masks). We also want to capture them through our own inference devices, which are equipped with an RGB camera, Infrared, and thermal sensors. Our project lead played a pivotal role in shaping the data collection process. Engaging in continuous discussions with our ML engineers, she acquired a precise understanding of the data we need, concluding that two designs are required.
Designing The Collecting Tool
The right tool is crucial for collecting good data. A good alignment of the training images and the data that the service receives when going online is ideal. For our event, we developed a special app. This app was designed not just to take photos, but also to help organize them efficiently with tags, making the process more streamlined end-to-end.
Designing the Event Traffic Flow
The traffic flow during the event is the second design our project lead had to pay attention to. To maximize efficiency, she decided to take a "Free flow" style instead of a "Linear flow" style.
"Going linear is much more organized and it helps us to keep track of each participant's progress easily," she said, "but the waiting time also increases if we can't precisely control the time consumption of each collecting stage, and I rather have time used on collecting data than waiting in line."
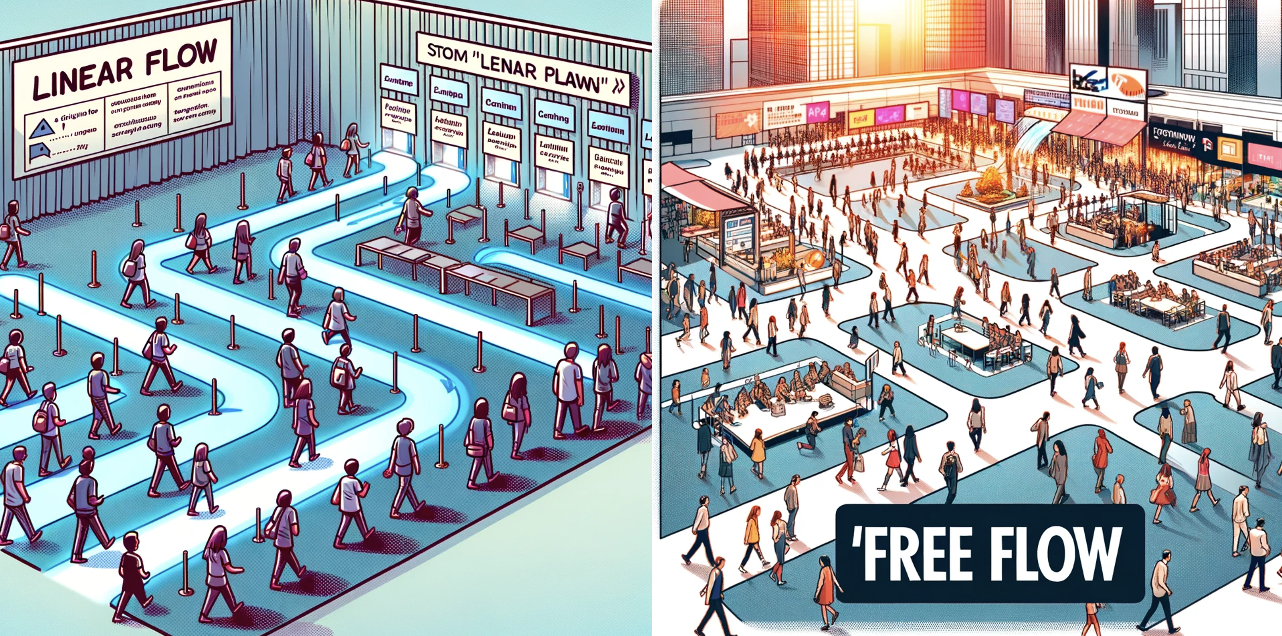
Although we considered the design of the traffic flow early in our planning, it's often common to address this after the next two crucial steps. One of these steps, which is widely regarded as the most challenging aspect of the project, is finding the right participants.
2. Finding Participants
Securing the right participants emerged as our next significant hurdle. As a face recognition technology company, this entails compensating individuals willing to have their pictures taken for our project. We assured participants that their data would be strictly used for training and benchmarking purposes and would remain confined within the company's database.
Our project lead weighed in on the decision-making process: 'There are two paths we could take for this task,' she noted, 'but realistically, only one is feasible.' The options before us were as follows:
Option 1: Working with a Vendor
- (Pros)Vendors bring trust and experience: they have a reputation on how to make events work
- (Pros)They can help find the right participants.
- (Pros)They handle legal aspects effectively.
- (Pros)They can even provide workers that will handle most of the non-technical tasks for us.
- (Cons)Higher costs and more energy are needed for coordination.
Option 2: Finding Participants Ourselves
On the other hand, doing it yourself is more challenging and time-consuming, with potential legal and quality issues. Be ready to meet problems like:
- Not enough participants found
- Participants not showing up or not cooperating
- Contracts with the participants need to be reviewed early
- Managing participants’ consent (like the complexities found in GDPR)
The estimation of cost and risk easily surpasses the other option, leaving us with an easy choice.
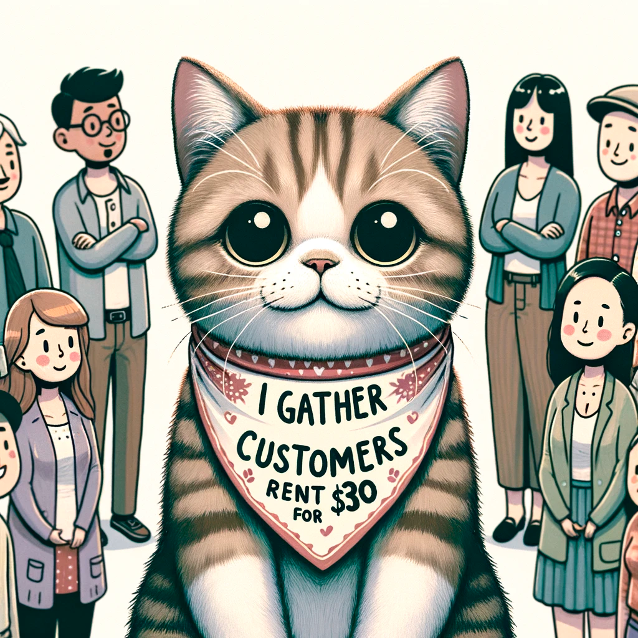
3. Creating the Scenario
Our objective was to ensure that our data mirrored real use case scenarios as closely as possible. This meant designing our data collection scenarios with these real-life applications in mind, taking into account factors such as background, lighting, the distance between the participant and the capture device, and demographic variables like age and gender.
However, capturing a comprehensive range of scenarios in a single event takes a lot of work. Therefore, it became crucial for our project lead to identify and prioritize scenarios that were most relevant and impactful. This involved balancing various elements: the budget, the time available, the limitations of our event space, and the practicalities of real-world conditions.
Word After the Event
The project lead faced a range of factors and challenges, testing her diverse skill set. When asked about the most important skill for hosting a successful event, she emphasized, “Imagination is key. It's about communicating with stakeholders, understanding their needs, and creatively envisioning the data collection scenarios. We try to imagine every possibility – how participants might interact with the setup, how different lighting conditions might affect the outcome, and what could potentially confuse participants or disrupt the process.”
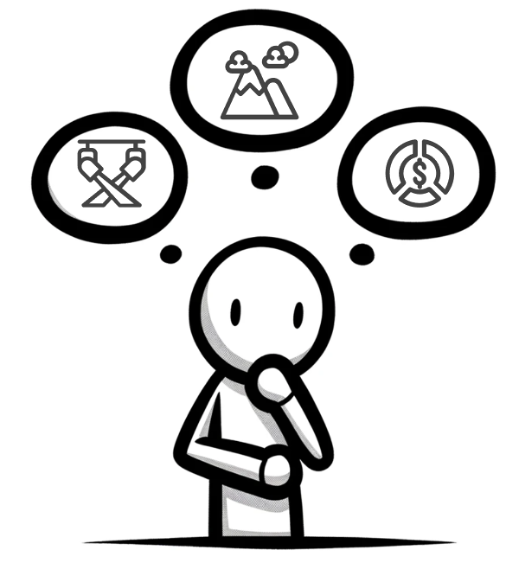
She added with a smile, “This time, our focus was on minimizing waiting time and maintaining flexibility, given our limited number of image collection devices and the need to gather a substantial amount of data. Thankfully, everything went according to plan.”
The event took place on 12/2023 and collected enough images for Japan Computer Vision to train a next-generation liveness detection AI model.
In our next blog, we’ll look at what happens to the data after it’s collected, exploring the crucial role of data scientists in this process. Stay tuned!